In a previous post, I discussed how 3D software could improve the resolution of Bible maps by fractally enhancing a digital elevation model and then synthetically creating landcover. In this post I’ll look at how machine learning can increase the resolution of freely available satellite images to generate realistic-looking historical maps.
Acquiring satellite imagery
The European Sentinel-2 satellites take daily photos of much of the earth at a ten-meter optical resolution (i.e., one pixel represents a ten-meter square on the ground). The U.S. operates a similar system, Landsat 8, with a fifteen-meter resolution. Commercial vendors offer much higher-resolution imagery, similar to what you find in Google Maps, at a prohibitive cost (thousands of dollars). By contrast, both Sentinel-2 and Landsat are government-operated and have freely available imagery. Here’s a comparison of the two, zoomed in to level 16 (1.3 meters per pixel), or well above their actual resolution:
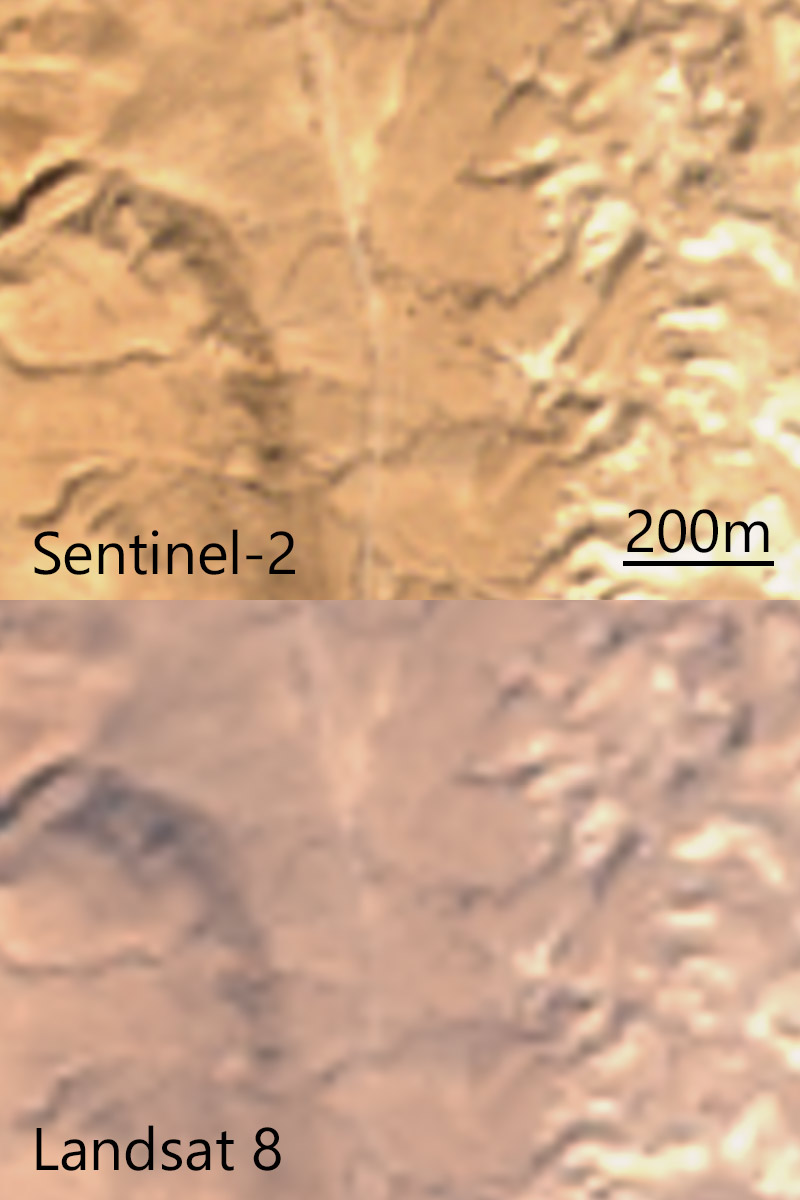
The Sentinel-2 imagery looks sharper thanks to its higher resolution, though the processing to correct the color overexposes the light areas, in my opinion. Because I want to start with the sharpest imagery, for this post I’ll use Sentinel-2.
I use Sentinel Playground to find a scene that doesn’t have a lot of clouds and then download the L2A, or atmosphere- and color-corrected, imagery. If I were producing a large-scale map that involved stitching together multiple photos, I’d use something like Sen2Agri to create a mosaic of many images, or a “basemap” (as in Google Maps). (Doing so is complicated and beyond the scope of this post.)
I choose a fourteen-kilometer-wide scene from January 2018 showing a mix of developed and undeveloped land near the northwest corner of the Dead Sea at a resolution of ten meters per pixel. I lower the gamma to 0.5 so that the colors approximately match the colors in Google Maps to allow for easier comparisons.
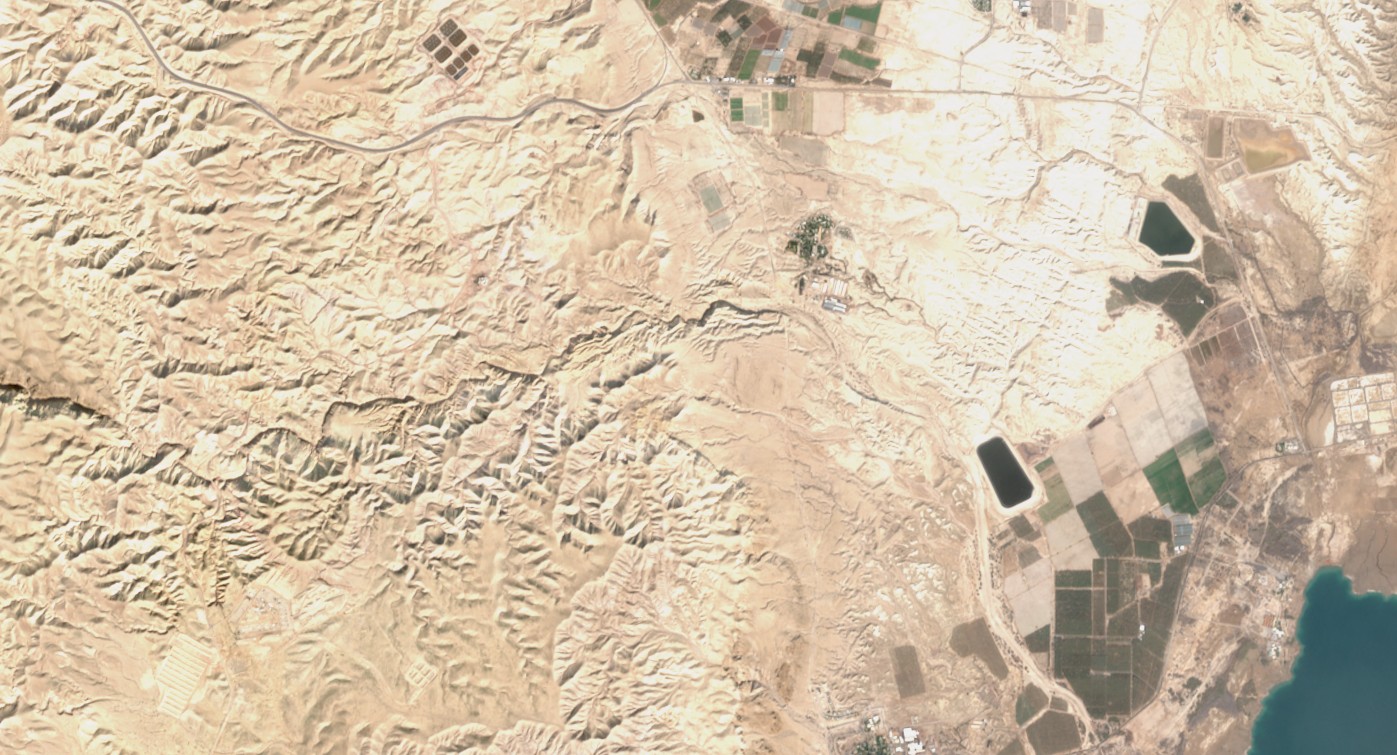
Increasing resolution
“Enhance!” is a staple of crime dramas, where a technician magically increases the resolution of a photo to provide crucial evidence needed by the plot. Super-resolution doesn’t work as well in reality as it does in fiction, but machine learning algorithms have increased in their sophistication in the past two years, and I thought it would be worth seeing how they performed on satellite photos. Here’s a detail of the above image, as enlarged by four different algorithms, plus Google Maps as the “ground truth.”
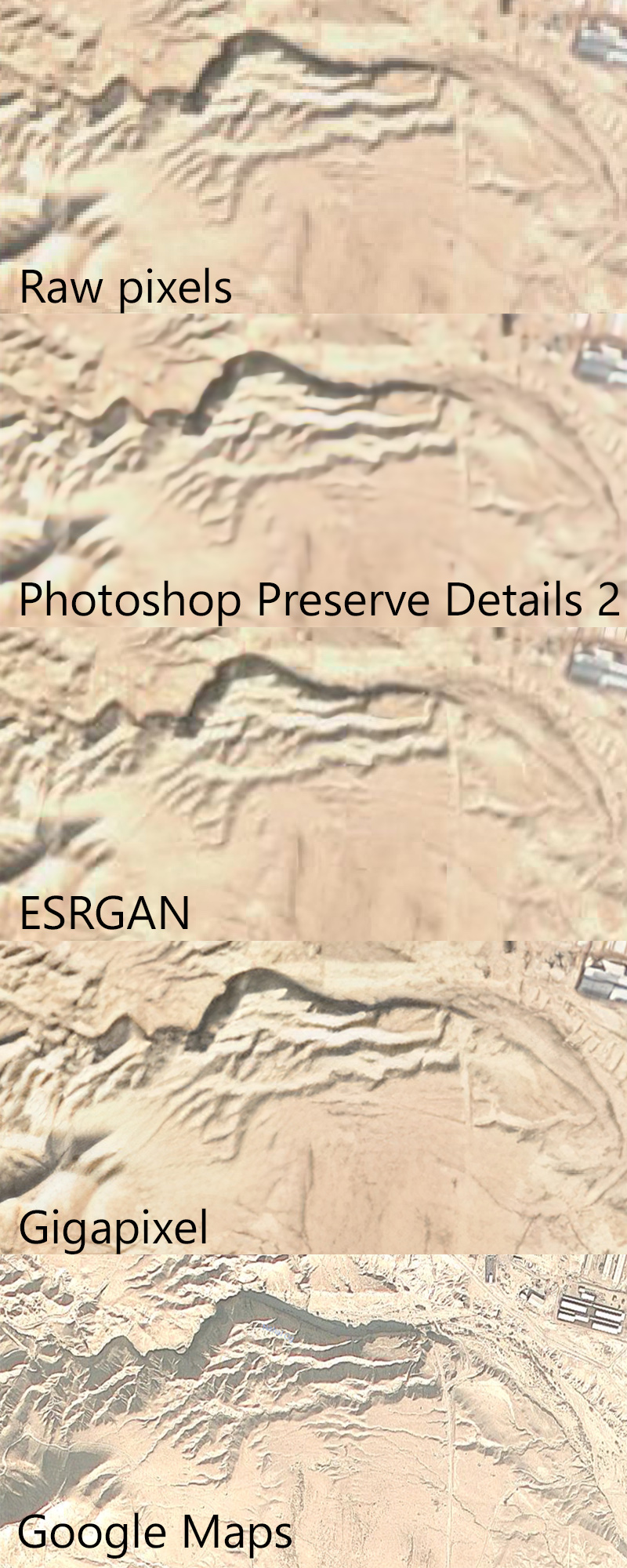
Each algorithm increases the original resolution by four times, providing a theoretical resolution of 2.5 meters per pixel.
The first, “raw pixels,” is the simplest; each pixel in the original image now occupies sixteen pixels (4×4). It was instantaneous to produce.
The second, “Photoshop Preserve Details 2.0,” uses the machine-learning algorithm built into recent versions of Photoshop. This algorithm took a few seconds to run. Generated image (1 MB).
The third, ESRGAN as implemented in Runway, reflects a state-of-the-art super-resolution algorithm for photos, though it’s not optimized for satellite imagery. This algorithm took about a minute to run on a “cloud GPU.” Generated image (1 MB).
The fourth, Gigapixel, uses a proprietary algorithm to sharpen photos; it also isn’t optimized for satellite imagery. This algorithm took about an hour to run on a CPU. Generated image (6 MB).
The fifth, Google Maps, reflects actual high-resolution (my guess is around 3.7 meters per pixel) photography.
Discussion
To my eye, the Gigapixel enlargement looks sharpest; it plausibly adds detail, though I don’t think anyone would mistake it for an actual 2.5-meter resolution satellite photo.
The stock ESRGAN enlargement doesn’t look quite as good to me; however, in my opinion, ESRGAN offers a lot of potential if tweaked. The algorithm already shows promise in upscaling video-game textures–a use the algorithm’s creators didn’t envision–and I think that taking the existing model developed by the researchers and training it further on satellite photos could produce higher-quality images.
I didn’t test the one purpose-built satellite image super-resolution algorithm I found because it’s designed for much-higher-resolution (thirty-centimeter) input imagery.
Removing modern features
One problem with using satellite photos as the base for historical maps involves dealing with modern features: agriculture, cities, roads, etc., that weren’t around in the same form in the time period the historical map is depicting. Machine learning presents a solution for this problem, as well; Photoshop’s content-aware fill allows you to select an area of an image for Photoshop to plausibly fill in with similar content. For example, here’s the Gigapixel-enlarged image with human-created features removed by content-aware fill:
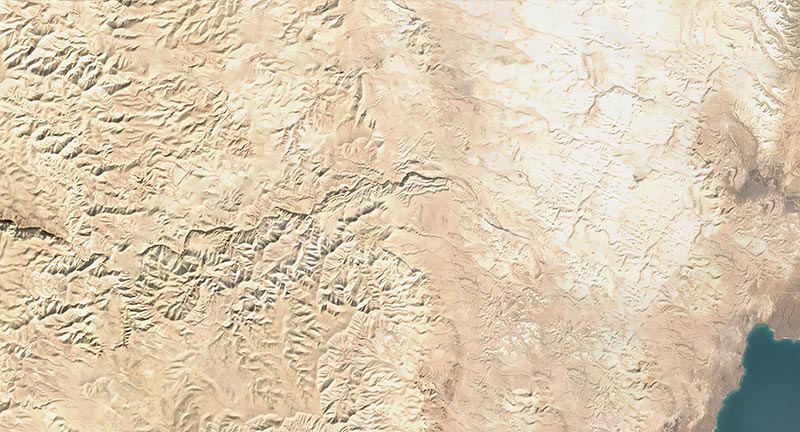
I made these edits by hand, but at scale you could use OpenStreetMap’s land-use data to mask candidate areas for content-aware replacement:
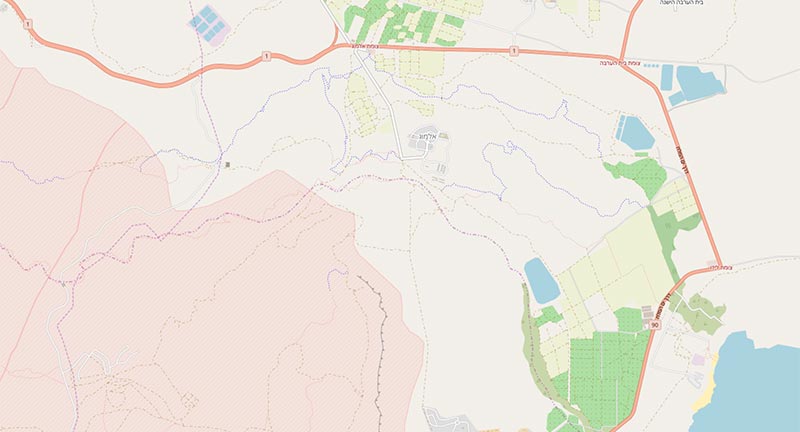
Conclusion
If you want to work with satellite imagery to produce a high-resolution basemap for historical or Bible maps, then using machine learning both to sharpen them and to remove modern features could be a viable, if time-consuming, process. The image in this post covers about 100 square kilometers; modern Israel is over 20,000 square kilometers. And this scene contains a mostly undeveloped area; large-scale cities are harder to erase with content-aware fill because there’s less surrounding wilderness for the algorithm to work with. But if you’re willing to put in the work, the result could be a free, plausibly realistic, reasonably detailed map over which you can overlay your own data.