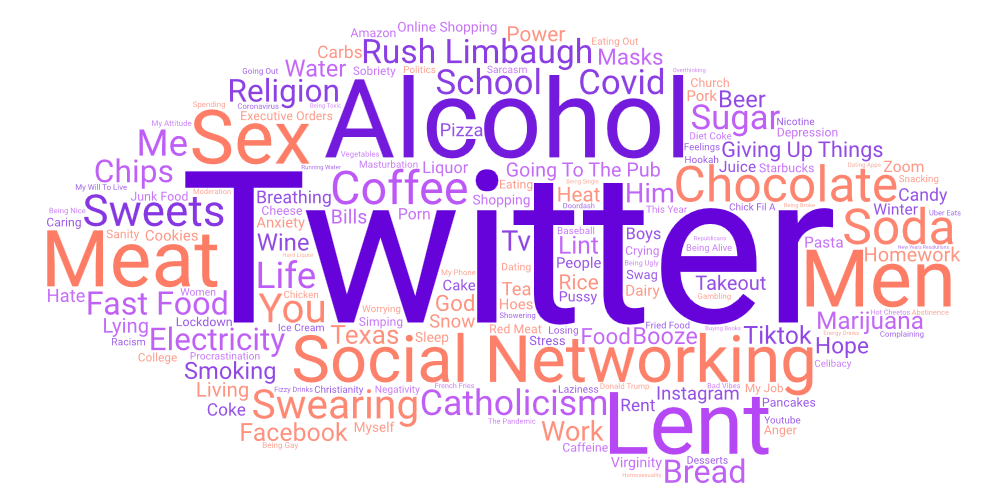
This year, the usual trio of Twitter, alcohol, and social networking led the list. But current events influenced the list this year more than most. Radio personality Rush Limbaugh‘s death on Ash Wednesday led many to comment that they were giving him up for Lent, landing him at #7. A major winter storm (#62, snow and #77, winter) in Texas (#34) knocked out electricity (#5), water (#35), and heat (#68) for millions of people. Finally, the global pandemic (#23, covid) elevated topics like going to the pub (#24) and suppressed others, like eating out (#75). Zoom (#63), a videoconferencing service, appeared for the first time. “Executive orders” (#53) refers to a satirical tweet about U.S. President Biden.
This year’s list draws from 24,312 tweets out of 557,560 total tweets mentioning Lent.
COVID-19
The global coronavirus pandemic led to jumps in several topics, most notably “going to the pub,” which was almost as popular as “COVID,” and was followed closely by food pickup and delivery.
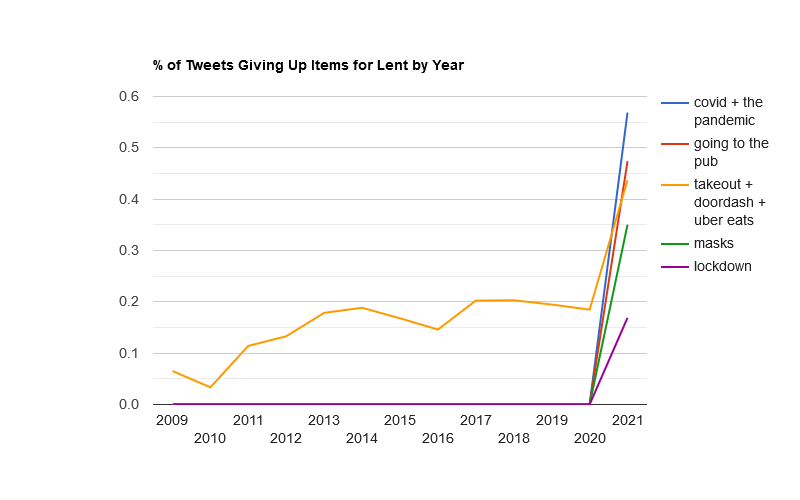
Social Media
In the battle of the social networks, TikTok has almost overtaken Instagram, while Snapchat fell below Tinder. Facebook, not shown on this chart because it makes the chart harder to read, was once dominant and is now only just above Instagram.
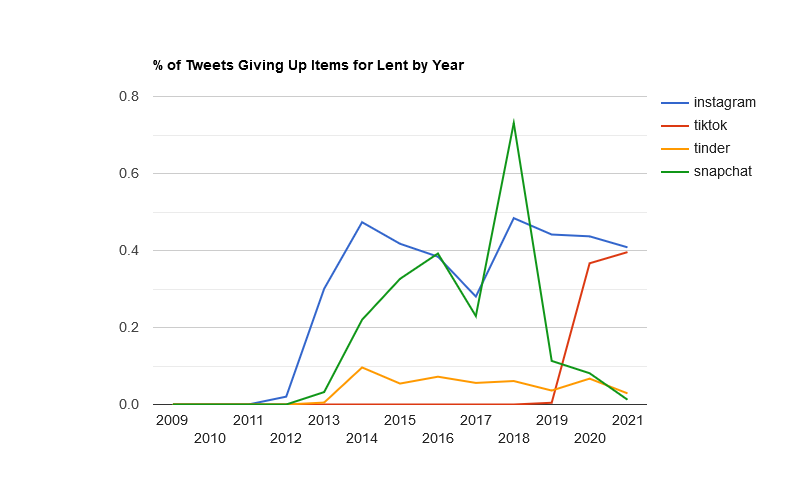
Fast Food
Fast food is down across the board.
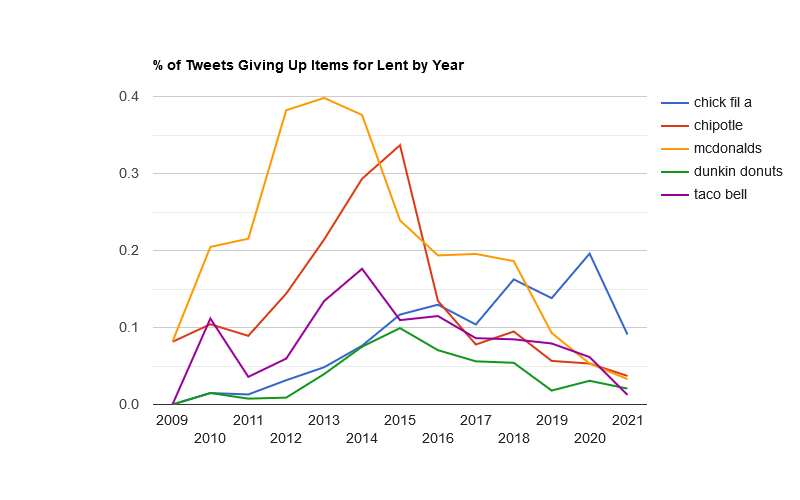
Top 103 Things Twitterers Gave Up for Lent in 2021
1. | 911 | +2 | |
2. | Alcohol | 895 | -1 |
3. | Social networking | 687 | -1 |
4. | Lent | 515 | +2 |
5. | Chocolate | 476 | 0 |
6. | Meat | 337 | -2 |
7. | Rush Limbaugh | 306 | |
8. | Swearing | 250 | +3 |
9. | Men | 242 | +4 |
10. | Sex | 225 | -2 |
11. | Coffee | 214 | -4 |
12. | Sweets | 213 | -2 |
13. | Giving up things | 188 | +14 |
14. | Catholicism | 185 | +9 |
15. | Electricity | 178 | +99 |
16. | Soda | 174 | -7 |
17. | Fast food | 167 | -5 |
18. | Religion | 154 | +6 |
19. | School | 140 | -2 |
20. | Marijuana | 138 | 0 |
21. | Sugar | 136 | +1 |
22. | Chips | 123 | -3 |
23. | Covid | 116 | |
24. | Going to the pub | 115 | |
25. | Bread | 115 | -7 |
26. | Smoking | 114 | +5 |
27. | 113 | +3 | |
28. | You | 111 | -13 |
29. | Work | 102 | -13 |
30. | Life | 99 | -2 |
31. | 99 | -2 | |
32. | Tiktok | 96 | +1 |
33. | Booze | 94 | +12 |
34. | Texas | 92 | |
35. | Water | 87 | +61 |
36. | Masks | 85 | |
37. | Online shopping | 85 | +24 |
38. | Homework | 83 | +5 |
39. | Hope | 82 | +15 |
40. | Living | 80 | +36 |
41. | Takeout | 77 | +26 |
42. | Breathing | 75 | +17 |
43. | Beer | 71 | -22 |
44. | Wine | 71 | -4 |
45. | Power | 61 | |
46. | Candy | 60 | -12 |
47. | Food | 59 | -1 |
48. | Him | 59 | +6 |
49. | Depression | 58 | -14 |
50. | Shopping | 55 | +7 |
51. | Lying | 53 | -3 |
52. | Executive orders | 53 | |
53. | Virginity | 51 | -28 |
54. | Liquor | 51 | -16 |
55. | Cookies | 51 | -2 |
56. | Starbucks | 51 | -26 |
57. | Red meat | 50 | -18 |
58. | Christianity | 49 | +18 |
59. | Junk food | 49 | -17 |
60. | Procrastination | 49 | 0 |
61. | Anxiety | 47 | -18 |
62. | Snow | 46 | +47 |
63. | Zoom | 44 | |
64. | Rice | 44 | -14 |
65. | College | 42 | -28 |
66. | Sobriety | 41 | -8 |
67. | Lockdown | 41 | |
68. | Heat | 40 | +44 |
69. | Carbs | 40 | -28 |
70. | Pancakes | 40 | +3 |
71. | Caffeine | 40 | -24 |
72. | Donald Trump | 40 | -22 |
73. | Masturbation | 39 | -24 |
74. | Desserts | 38 | -3 |
75. | Eating out | 38 | -22 |
76. | Simping | 36 | -34 |
77. | Winter | 36 | +28 |
78. | Ice cream | 34 | -14 |
79. | Lint | 34 | +1 |
80. | God | 33 | +5 |
81. | Cheese | 33 | -30 |
82. | Negativity | 33 | -22 |
83. | People | 32 | -34 |
84. | Complaining | 32 | -28 |
85. | Being alive | 32 | +19 |
86. | Church | 31 | -16 |
87. | Coke | 29 | -25 |
88. | Pussy | 28 | -22 |
89. | Boys | 28 | -57 |
90. | Pizza | 28 | -29 |
91. | Sleep | 28 | -8 |
92. | My will to live | 28 | -11 |
93. | Amazon | 27 | -1 |
94. | Sarcasm | 26 | -5 |
95. | Hate | 26 | +15 |
96. | Snacking | 25 | -15 |
97. | Sanity | 24 | 0 |
98. | My job | 24 | -44 |
99. | Cake | 23 | -22 |
100. | Dairy | 22 | -56 |
101. | The pandemic | 22 | |
102. | Chick Fil A | 22 | -47 |
103. | Baseball | 22 | +9 |
Top Categories
1. | food | 3,419 |
2. | technology | 2,305 |
3. | smoking/drugs/alcohol | 1,613 |
4. | irony | 1,341 |
5. | habits | 1,198 |
6. | relationship | 804 |
7. | religion | 528 |
8. | sex | 476 |
9. | health/hygiene | 442 |
10. | school/work | 414 |
11. | celebrity | 313 |
12. | politics | 242 |
13. | weather | 213 |
14. | shopping | 172 |
15. | money | 125 |
16. | entertainment | 106 |
17. | sports | 48 |
18. | clothes | 15 |
19. | possessions | 12 |